This notebook is from the Hands on Machine Learning using Scikit-Learn and Tensorflow 2, 2nd edition
|
Time Series Prediction using RNNs#
First, let’s import a few common modules, ensure MatplotLib plots figures inline and prepare a function to save the figures. We also check that Python 3.5 or later is installed (although Python 2.x may work, it is deprecated so we strongly recommend you use Python 3 instead), as well as Scikit-Learn ≥0.20 and TensorFlow ≥2.0.
# Python ≥3.5 is required
import sys
assert sys.version_info >= (3, 5)
# Is this notebook running on Colab or Kaggle?
IS_COLAB = "google.colab" in sys.modules
IS_KAGGLE = "kaggle_secrets" in sys.modules
# Scikit-Learn ≥0.20 is required
import sklearn
assert sklearn.__version__ >= "0.20"
# TensorFlow ≥2.0 is required
import tensorflow as tf
from tensorflow import keras
assert tf.__version__ >= "2.0"
if not tf.config.list_physical_devices('GPU'):
print("No GPU was detected. LSTMs and CNNs can be very slow without a GPU.")
if IS_COLAB:
print("Go to Runtime > Change runtime and select a GPU hardware accelerator.")
if IS_KAGGLE:
print("Go to Settings > Accelerator and select GPU.")
# Common imports
import numpy as np
import os
from pathlib import Path
# to make this notebook's output stable across runs
np.random.seed(42)
tf.random.set_seed(42)
# To plot pretty figures
%matplotlib inline
import matplotlib as mpl
import matplotlib.pyplot as plt
mpl.rc('axes', labelsize=14)
mpl.rc('xtick', labelsize=12)
mpl.rc('ytick', labelsize=12)
# Where to save the figures
PROJECT_ROOT_DIR = "."
CHAPTER_ID = "rnn"
IMAGES_PATH = os.path.join(PROJECT_ROOT_DIR, "images", CHAPTER_ID)
os.makedirs(IMAGES_PATH, exist_ok=True)
def save_fig(fig_id, tight_layout=True, fig_extension="png", resolution=300):
path = os.path.join(IMAGES_PATH, fig_id + "." + fig_extension)
print("Saving figure", fig_id)
if tight_layout:
plt.tight_layout()
plt.savefig(path, format=fig_extension, dpi=resolution)
Basic RNNs#
Generate the Dataset#
def generate_time_series(batch_size, n_steps):
freq1, freq2, offsets1, offsets2 = np.random.rand(4, batch_size, 1)
time = np.linspace(0, 1, n_steps)
series = 0.5 * np.sin((time - offsets1) * (freq1 * 10 + 10)) # wave 1
series += 0.2 * np.sin((time - offsets2) * (freq2 * 20 + 20)) # + wave 2
series += 0.1 * (np.random.rand(batch_size, n_steps) - 0.5) # + noise
return series[..., np.newaxis].astype(np.float32)
np.random.seed(42)
n_steps = 50
series = generate_time_series(10000, n_steps + 1)
X_train, y_train = series[:7000, :n_steps], series[:7000, -1]
X_valid, y_valid = series[7000:9000, :n_steps], series[7000:9000, -1]
X_test, y_test = series[9000:, :n_steps], series[9000:, -1]
X_train.shape, y_train.shape
((7000, 50, 1), (7000, 1))
def plot_series(series, y=None, y_pred=None, x_label="$t$", y_label="$x(t)$", legend=True):
plt.plot(series, ".-")
if y is not None:
plt.plot(n_steps, y, "bo", label="Target")
if y_pred is not None:
plt.plot(n_steps, y_pred, "rx", markersize=10, label="Prediction")
plt.grid(True)
if x_label:
plt.xlabel(x_label, fontsize=16)
if y_label:
plt.ylabel(y_label, fontsize=16, rotation=0)
plt.hlines(0, 0, 100, linewidth=1)
plt.axis([0, n_steps + 1, -1, 1])
if legend and (y or y_pred):
plt.legend(fontsize=14, loc="upper left")
fig, axes = plt.subplots(nrows=1, ncols=3, sharey=True, figsize=(12, 4))
for col in range(3):
plt.sca(axes[col])
plot_series(X_valid[col, :, 0], y_valid[col, 0],
y_label=("$x(t)$" if col==0 else None),
legend=(col == 0))
save_fig("time_series_plot")
plt.show()
Saving figure time_series_plot
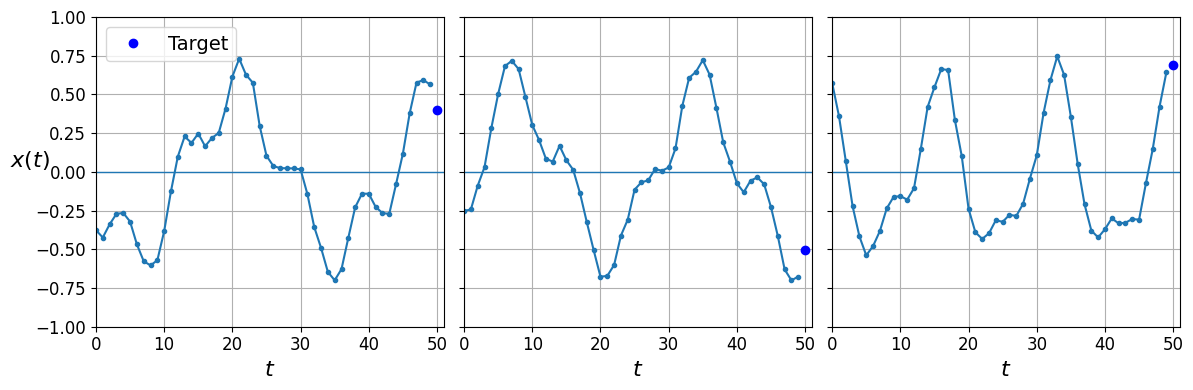
Note: in this notebook, the blue dots represent targets, and red crosses represent predictions. In the book, I first used blue crosses for targets and red dots for predictions, then I reversed this later in the chapter. Sorry if this caused some confusion.
Computing Some Baselines#
Naive predictions (just predict the last observed value):
y_pred = X_valid[:, -1]
np.mean(keras.losses.mean_squared_error(y_valid, y_pred))
2023-04-04 13:03:42.738268: I tensorflow/compiler/xla/stream_executor/cuda/cuda_gpu_executor.cc:996] successful NUMA node read from SysFS had negative value (-1), but there must be at least one NUMA node, so returning NUMA node zero. See more at https://github.com/torvalds/linux/blob/v6.0/Documentation/ABI/testing/sysfs-bus-pci#L344-L355
2023-04-04 13:03:42.738469: I tensorflow/compiler/xla/stream_executor/cuda/cuda_gpu_executor.cc:996] successful NUMA node read from SysFS had negative value (-1), but there must be at least one NUMA node, so returning NUMA node zero. See more at https://github.com/torvalds/linux/blob/v6.0/Documentation/ABI/testing/sysfs-bus-pci#L344-L355
2023-04-04 13:03:42.738547: I tensorflow/compiler/xla/stream_executor/cuda/cuda_gpu_executor.cc:996] successful NUMA node read from SysFS had negative value (-1), but there must be at least one NUMA node, so returning NUMA node zero. See more at https://github.com/torvalds/linux/blob/v6.0/Documentation/ABI/testing/sysfs-bus-pci#L344-L355
2023-04-04 13:03:45.573522: I tensorflow/compiler/xla/stream_executor/cuda/cuda_gpu_executor.cc:996] successful NUMA node read from SysFS had negative value (-1), but there must be at least one NUMA node, so returning NUMA node zero. See more at https://github.com/torvalds/linux/blob/v6.0/Documentation/ABI/testing/sysfs-bus-pci#L344-L355
2023-04-04 13:03:45.573896: I tensorflow/compiler/xla/stream_executor/cuda/cuda_gpu_executor.cc:996] successful NUMA node read from SysFS had negative value (-1), but there must be at least one NUMA node, so returning NUMA node zero. See more at https://github.com/torvalds/linux/blob/v6.0/Documentation/ABI/testing/sysfs-bus-pci#L344-L355
2023-04-04 13:03:45.574148: I tensorflow/compiler/xla/stream_executor/cuda/cuda_gpu_executor.cc:996] successful NUMA node read from SysFS had negative value (-1), but there must be at least one NUMA node, so returning NUMA node zero. See more at https://github.com/torvalds/linux/blob/v6.0/Documentation/ABI/testing/sysfs-bus-pci#L344-L355
2023-04-04 13:03:45.574349: I tensorflow/core/common_runtime/gpu/gpu_device.cc:1635] Created device /job:localhost/replica:0/task:0/device:GPU:0 with 13868 MB memory: -> device: 0, name: NVIDIA RTX A4500 Laptop GPU, pci bus id: 0000:01:00.0, compute capability: 8.6
0.020211367
plot_series(X_valid[0, :, 0], y_valid[0, 0], y_pred[0, 0])
plt.show()
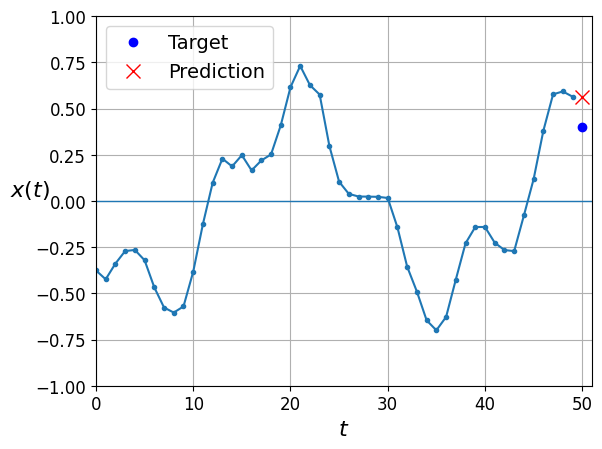
Linear predictions:
np.random.seed(42)
tf.random.set_seed(42)
model = keras.models.Sequential([
keras.layers.Flatten(input_shape=[50, 1]),
keras.layers.Dense(1)
])
optimizer=tf.keras.optimizers.legacy.Adam()
model.compile(loss="mse", optimizer=optimizer)
history = model.fit(X_train, y_train, epochs=20,
validation_data=(X_valid, y_valid))
Epoch 1/20
219/219 [==============================] - 2s 3ms/step - loss: 0.1313 - val_loss: 0.0564
Epoch 2/20
219/219 [==============================] - 0s 2ms/step - loss: 0.0298 - val_loss: 0.0183
Epoch 3/20
219/219 [==============================] - 0s 2ms/step - loss: 0.0132 - val_loss: 0.0106
Epoch 4/20
219/219 [==============================] - 0s 2ms/step - loss: 0.0091 - val_loss: 0.0082
Epoch 5/20
219/219 [==============================] - 1s 2ms/step - loss: 0.0074 - val_loss: 0.0071
Epoch 6/20
219/219 [==============================] - 0s 2ms/step - loss: 0.0065 - val_loss: 0.0063
Epoch 7/20
219/219 [==============================] - 0s 2ms/step - loss: 0.0059 - val_loss: 0.0057
Epoch 8/20
219/219 [==============================] - 0s 2ms/step - loss: 0.0054 - val_loss: 0.0052
Epoch 9/20
219/219 [==============================] - 0s 1ms/step - loss: 0.0050 - val_loss: 0.0049
Epoch 10/20
219/219 [==============================] - 0s 1ms/step - loss: 0.0047 - val_loss: 0.0046
Epoch 11/20
219/219 [==============================] - 0s 2ms/step - loss: 0.0045 - val_loss: 0.0044
Epoch 12/20
219/219 [==============================] - 0s 1ms/step - loss: 0.0043 - val_loss: 0.0043
Epoch 13/20
219/219 [==============================] - 0s 1ms/step - loss: 0.0042 - val_loss: 0.0040
Epoch 14/20
219/219 [==============================] - 0s 2ms/step - loss: 0.0041 - val_loss: 0.0039
Epoch 15/20
219/219 [==============================] - 0s 2ms/step - loss: 0.0040 - val_loss: 0.0038
Epoch 16/20
219/219 [==============================] - 0s 1ms/step - loss: 0.0039 - val_loss: 0.0040
Epoch 17/20
219/219 [==============================] - 0s 1ms/step - loss: 0.0038 - val_loss: 0.0037
Epoch 18/20
219/219 [==============================] - 0s 1ms/step - loss: 0.0038 - val_loss: 0.0037
Epoch 19/20
219/219 [==============================] - 0s 1ms/step - loss: 0.0037 - val_loss: 0.0037
Epoch 20/20
219/219 [==============================] - 0s 2ms/step - loss: 0.0037 - val_loss: 0.0036
model.evaluate(X_valid, y_valid)
63/63 [==============================] - 0s 793us/step - loss: 0.0036
0.003601563163101673
def plot_learning_curves(loss, val_loss):
plt.plot(np.arange(len(loss)) + 0.5, loss, "b.-", label="Training loss")
plt.plot(np.arange(len(val_loss)) + 1, val_loss, "r.-", label="Validation loss")
plt.gca().xaxis.set_major_locator(mpl.ticker.MaxNLocator(integer=True))
plt.axis([1, 20, 0, 0.05])
plt.legend(fontsize=14)
plt.xlabel("Epochs")
plt.ylabel("Loss")
plt.grid(True)
plot_learning_curves(history.history["loss"], history.history["val_loss"])
plt.show()
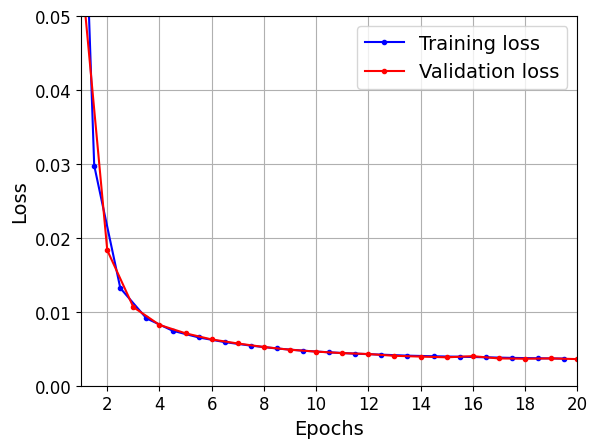
y_pred = model.predict(X_valid)
plot_series(X_valid[0, :, 0], y_valid[0, 0], y_pred[0, 0])
plt.show()
63/63 [==============================] - 0s 586us/step
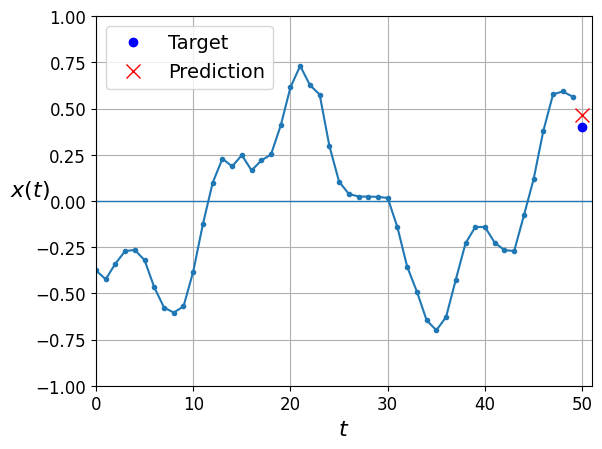
Using a Simple RNN#
np.random.seed(42)
tf.random.set_seed(42)
model = keras.models.Sequential([
keras.layers.SimpleRNN(1, input_shape=[None, 1])
])
optimizer=tf.keras.optimizers.legacy.Adam(learning_rate=0.005)
model.compile(loss="mse", optimizer=optimizer)
history = model.fit(X_train, y_train, epochs=20, validation_data=(X_valid, y_valid))
Epoch 1/20
219/219 [==============================] - 3s 11ms/step - loss: 0.1699 - val_loss: 0.1090
Epoch 2/20
219/219 [==============================] - 3s 12ms/step - loss: 0.0630 - val_loss: 0.0332
Epoch 3/20
219/219 [==============================] - 2s 11ms/step - loss: 0.0196 - val_loss: 0.0132
Epoch 4/20
219/219 [==============================] - 2s 11ms/step - loss: 0.0122 - val_loss: 0.0114
Epoch 5/20
219/219 [==============================] - 2s 10ms/step - loss: 0.0117 - val_loss: 0.0112
Epoch 6/20
219/219 [==============================] - 3s 12ms/step - loss: 0.0116 - val_loss: 0.0111
Epoch 7/20
219/219 [==============================] - 2s 11ms/step - loss: 0.0115 - val_loss: 0.0110
Epoch 8/20
219/219 [==============================] - 3s 12ms/step - loss: 0.0114 - val_loss: 0.0109
Epoch 9/20
219/219 [==============================] - 2s 10ms/step - loss: 0.0114 - val_loss: 0.0109
Epoch 10/20
219/219 [==============================] - 2s 10ms/step - loss: 0.0114 - val_loss: 0.0109
Epoch 11/20
219/219 [==============================] - 2s 11ms/step - loss: 0.0114 - val_loss: 0.0109
Epoch 12/20
219/219 [==============================] - 3s 13ms/step - loss: 0.0114 - val_loss: 0.0109
Epoch 13/20
219/219 [==============================] - 3s 13ms/step - loss: 0.0114 - val_loss: 0.0109
Epoch 14/20
219/219 [==============================] - 2s 11ms/step - loss: 0.0114 - val_loss: 0.0109
Epoch 15/20
219/219 [==============================] - 3s 12ms/step - loss: 0.0114 - val_loss: 0.0109
Epoch 16/20
219/219 [==============================] - 3s 12ms/step - loss: 0.0114 - val_loss: 0.0109
Epoch 17/20
219/219 [==============================] - 3s 12ms/step - loss: 0.0114 - val_loss: 0.0109
Epoch 18/20
219/219 [==============================] - 3s 12ms/step - loss: 0.0114 - val_loss: 0.0109
Epoch 19/20
219/219 [==============================] - 3s 13ms/step - loss: 0.0114 - val_loss: 0.0109
Epoch 20/20
219/219 [==============================] - 3s 12ms/step - loss: 0.0114 - val_loss: 0.0109
model.evaluate(X_valid, y_valid)
63/63 [==============================] - 0s 6ms/step - loss: 0.0109
0.01088138297200203
plot_learning_curves(history.history["loss"], history.history["val_loss"])
plt.show()
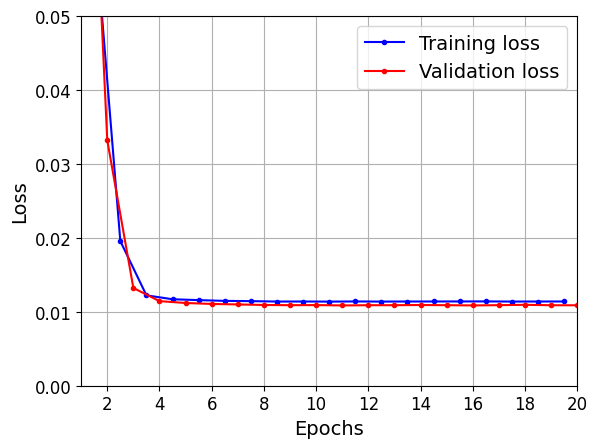
y_pred = model.predict(X_valid)
plot_series(X_valid[0, :, 0], y_valid[0, 0], y_pred[0, 0])
plt.show()
63/63 [==============================] - 1s 7ms/step
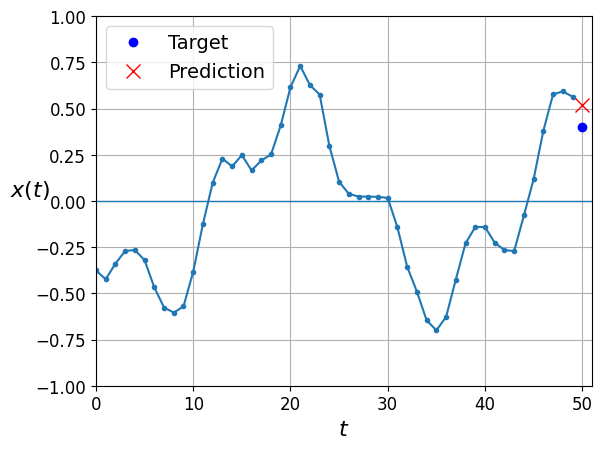
Deep RNNs#
np.random.seed(42)
tf.random.set_seed(42)
model = keras.models.Sequential([
keras.layers.SimpleRNN(20, return_sequences=True, input_shape=[None, 1]),
keras.layers.SimpleRNN(20, return_sequences=True),
keras.layers.SimpleRNN(1)
])
optimizer=tf.keras.optimizers.legacy.Adam()
model.compile(loss="mse", optimizer=optimizer)
history = model.fit(X_train, y_train, epochs=20,
validation_data=(X_valid, y_valid))
Epoch 1/20
5/219 [..............................] - ETA: 7s - loss: 0.2693 219/219 [==============================] - 9s 37ms/step - loss: 0.0254 - val_loss: 0.0053
Epoch 2/20
219/219 [==============================] - 8s 34ms/step - loss: 0.0045 - val_loss: 0.0036
Epoch 3/20
219/219 [==============================] - 8s 37ms/step - loss: 0.0034 - val_loss: 0.0030
Epoch 4/20
219/219 [==============================] - 8s 35ms/step - loss: 0.0032 - val_loss: 0.0030
Epoch 5/20
219/219 [==============================] - 8s 36ms/step - loss: 0.0030 - val_loss: 0.0030
Epoch 6/20
219/219 [==============================] - 8s 36ms/step - loss: 0.0030 - val_loss: 0.0028
Epoch 7/20
219/219 [==============================] - 8s 34ms/step - loss: 0.0029 - val_loss: 0.0028
Epoch 8/20
219/219 [==============================] - 8s 35ms/step - loss: 0.0030 - val_loss: 0.0029
Epoch 9/20
219/219 [==============================] - 8s 36ms/step - loss: 0.0029 - val_loss: 0.0026
Epoch 10/20
219/219 [==============================] - 8s 36ms/step - loss: 0.0028 - val_loss: 0.0027
Epoch 11/20
219/219 [==============================] - 8s 36ms/step - loss: 0.0028 - val_loss: 0.0026
Epoch 12/20
219/219 [==============================] - 8s 35ms/step - loss: 0.0028 - val_loss: 0.0027
Epoch 13/20
219/219 [==============================] - 8s 35ms/step - loss: 0.0028 - val_loss: 0.0027
Epoch 14/20
219/219 [==============================] - 8s 35ms/step - loss: 0.0028 - val_loss: 0.0028
Epoch 15/20
219/219 [==============================] - 8s 35ms/step - loss: 0.0028 - val_loss: 0.0031
Epoch 16/20
219/219 [==============================] - 8s 35ms/step - loss: 0.0029 - val_loss: 0.0026
Epoch 17/20
219/219 [==============================] - 8s 35ms/step - loss: 0.0027 - val_loss: 0.0026
Epoch 18/20
219/219 [==============================] - 8s 36ms/step - loss: 0.0027 - val_loss: 0.0025
Epoch 19/20
219/219 [==============================] - 8s 36ms/step - loss: 0.0027 - val_loss: 0.0026
Epoch 20/20
219/219 [==============================] - 8s 35ms/step - loss: 0.0027 - val_loss: 0.0025
2023-04-04 13:04:50.263686: I tensorflow/compiler/xla/stream_executor/cuda/cuda_blas.cc:637] TensorFloat-32 will be used for the matrix multiplication. This will only be logged once.
model.evaluate(X_valid, y_valid)
63/63 [==============================] - 1s 13ms/step - loss: 0.0025
0.0025295214727520943
plot_learning_curves(history.history["loss"], history.history["val_loss"])
plt.show()
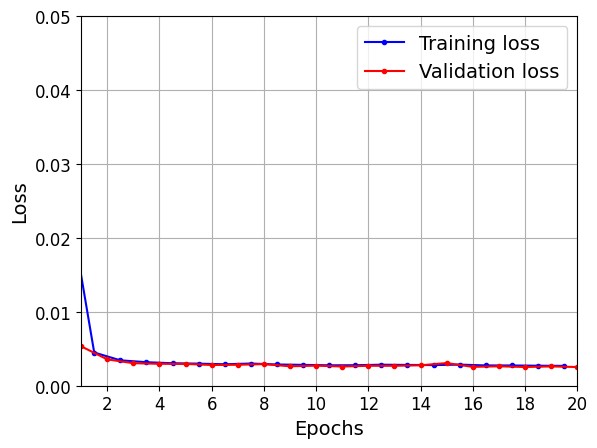
y_pred = model.predict(X_valid)
plot_series(X_valid[0, :, 0], y_valid[0, 0], y_pred[0, 0])
plt.show()
63/63 [==============================] - 1s 11ms/step
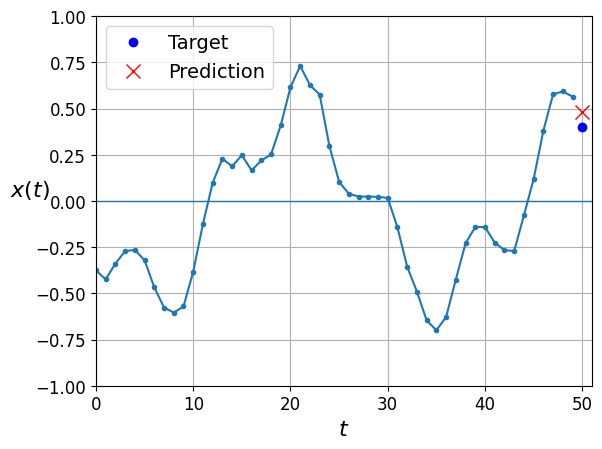
Make the second SimpleRNN
layer return only the last output:
np.random.seed(42)
tf.random.set_seed(42)
model = keras.models.Sequential([
keras.layers.SimpleRNN(20, return_sequences=True, input_shape=[None, 1]),
keras.layers.SimpleRNN(20),
keras.layers.Dense(1)
])
optimizer=tf.keras.optimizers.legacy.Adam()
model.compile(loss="mse", optimizer=optimizer)
history = model.fit(X_train, y_train, epochs=20,
validation_data=(X_valid, y_valid))
Epoch 1/20
219/219 [==============================] - 6s 25ms/step - loss: 0.0225 - val_loss: 0.0055
Epoch 2/20
219/219 [==============================] - 5s 25ms/step - loss: 0.0045 - val_loss: 0.0039
Epoch 3/20
219/219 [==============================] - 5s 25ms/step - loss: 0.0034 - val_loss: 0.0030
Epoch 4/20
219/219 [==============================] - 5s 24ms/step - loss: 0.0031 - val_loss: 0.0030
Epoch 5/20
219/219 [==============================] - 5s 24ms/step - loss: 0.0030 - val_loss: 0.0031
Epoch 6/20
219/219 [==============================] - 5s 25ms/step - loss: 0.0030 - val_loss: 0.0027
Epoch 7/20
219/219 [==============================] - 5s 25ms/step - loss: 0.0029 - val_loss: 0.0030
Epoch 8/20
219/219 [==============================] - 5s 24ms/step - loss: 0.0030 - val_loss: 0.0027
Epoch 9/20
219/219 [==============================] - 5s 24ms/step - loss: 0.0029 - val_loss: 0.0026
Epoch 10/20
219/219 [==============================] - 5s 24ms/step - loss: 0.0028 - val_loss: 0.0027
Epoch 11/20
219/219 [==============================] - 5s 25ms/step - loss: 0.0028 - val_loss: 0.0027
Epoch 12/20
219/219 [==============================] - 5s 24ms/step - loss: 0.0028 - val_loss: 0.0027
Epoch 13/20
219/219 [==============================] - 5s 25ms/step - loss: 0.0028 - val_loss: 0.0028
Epoch 14/20
219/219 [==============================] - 5s 25ms/step - loss: 0.0028 - val_loss: 0.0027
Epoch 15/20
219/219 [==============================] - 5s 24ms/step - loss: 0.0029 - val_loss: 0.0027
Epoch 16/20
219/219 [==============================] - 5s 25ms/step - loss: 0.0028 - val_loss: 0.0026
Epoch 17/20
219/219 [==============================] - 5s 24ms/step - loss: 0.0028 - val_loss: 0.0027
Epoch 18/20
219/219 [==============================] - 5s 24ms/step - loss: 0.0028 - val_loss: 0.0026
Epoch 19/20
219/219 [==============================] - 5s 25ms/step - loss: 0.0027 - val_loss: 0.0027
Epoch 20/20
219/219 [==============================] - 5s 24ms/step - loss: 0.0027 - val_loss: 0.0026
model.evaluate(X_valid, y_valid)
63/63 [==============================] - 1s 11ms/step - loss: 0.0026
0.0025954328011721373
plot_learning_curves(history.history["loss"], history.history["val_loss"])
plt.show()
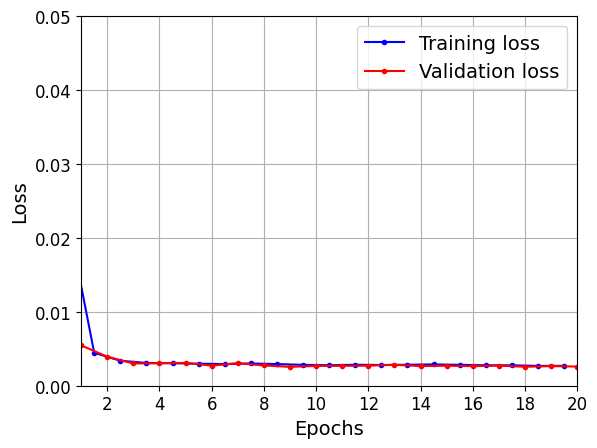
y_pred = model.predict(X_valid)
plot_series(X_valid[0, :, 0], y_valid[0, 0], y_pred[0, 0])
plt.show()
63/63 [==============================] - 1s 10ms/step
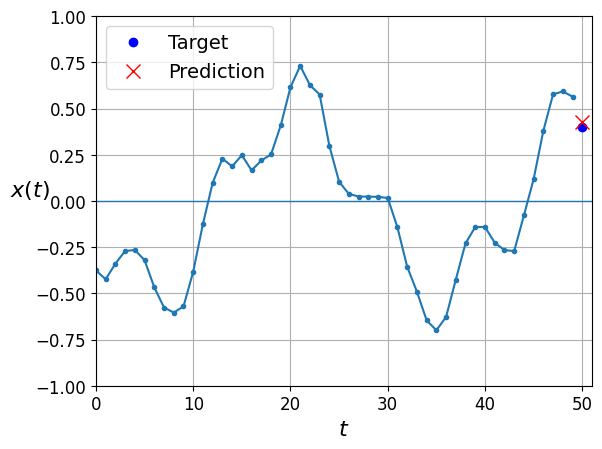
Forecasting Several Steps Ahead#
np.random.seed(43) # not 42, as it would give the first series in the train set
series = generate_time_series(1, n_steps + 10)
X_new, Y_new = series[:, :n_steps], series[:, n_steps:]
X = X_new
for step_ahead in range(10):
y_pred_one = model.predict(X[:, step_ahead:])[:, np.newaxis, :]
X = np.concatenate([X, y_pred_one], axis=1)
Y_pred = X[:, n_steps:]
1/1 [==============================] - 0s 18ms/step
1/1 [==============================] - 0s 18ms/step
1/1 [==============================] - 0s 22ms/step
1/1 [==============================] - 0s 18ms/step
1/1 [==============================] - 0s 18ms/step
1/1 [==============================] - 0s 22ms/step
1/1 [==============================] - 0s 19ms/step
1/1 [==============================] - 0s 19ms/step
1/1 [==============================] - 0s 21ms/step
1/1 [==============================] - 0s 20ms/step
Y_pred.shape
(1, 10, 1)
def plot_multiple_forecasts(X, Y, Y_pred):
n_steps = X.shape[1]
ahead = Y.shape[1]
plot_series(X[0, :, 0])
plt.plot(np.arange(n_steps, n_steps + ahead), Y[0, :, 0], "bo-", label="Actual")
plt.plot(np.arange(n_steps, n_steps + ahead), Y_pred[0, :, 0], "rx-", label="Forecast", markersize=10)
plt.axis([0, n_steps + ahead, -1, 1])
plt.legend(fontsize=14)
plot_multiple_forecasts(X_new, Y_new, Y_pred)
save_fig("forecast_ahead_plot")
plt.show()
Saving figure forecast_ahead_plot
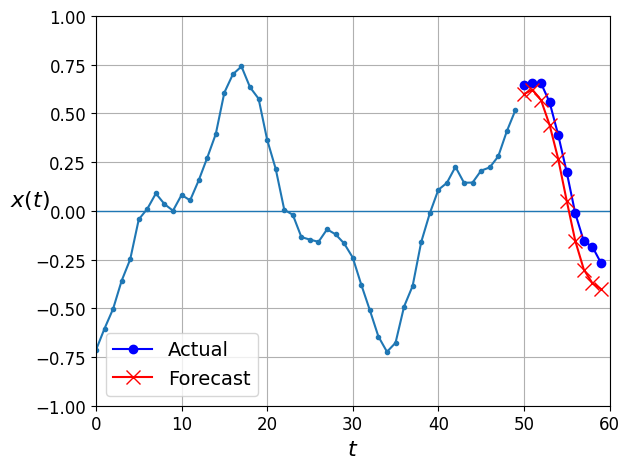
Now let’s use this model to predict the next 10 values. We first need to regenerate the sequences with 9 more time steps.
np.random.seed(42)
n_steps = 50
series = generate_time_series(10000, n_steps + 10)
X_train, Y_train = series[:7000, :n_steps], series[:7000, -10:, 0]
X_valid, Y_valid = series[7000:9000, :n_steps], series[7000:9000, -10:, 0]
X_test, Y_test = series[9000:, :n_steps], series[9000:, -10:, 0]
Now let’s predict the next 10 values one by one:
X = X_valid
for step_ahead in range(10):
y_pred_one = model.predict(X)[:, np.newaxis, :]
X = np.concatenate([X, y_pred_one], axis=1)
Y_pred = X[:, n_steps:, 0]
63/63 [==============================] - 1s 10ms/step
63/63 [==============================] - 1s 8ms/step
63/63 [==============================] - 0s 6ms/step
63/63 [==============================] - 0s 6ms/step
63/63 [==============================] - 1s 9ms/step
63/63 [==============================] - 1s 11ms/step
63/63 [==============================] - 1s 8ms/step
63/63 [==============================] - 0s 7ms/step
63/63 [==============================] - 0s 6ms/step
63/63 [==============================] - 0s 5ms/step
Y_pred.shape
(2000, 10)
np.mean(keras.metrics.mean_squared_error(Y_valid, Y_pred))
0.021216832
Let’s compare this performance with some baselines: naive predictions and a simple linear model:
Y_naive_pred = np.tile(X_valid[:, -1], 10) # take the last time step value, and repeat it 10 times
np.mean(keras.metrics.mean_squared_error(Y_valid, Y_naive_pred))
0.25697407
np.random.seed(42)
tf.random.set_seed(42)
model = keras.models.Sequential([
keras.layers.Flatten(input_shape=[50, 1]),
keras.layers.Dense(10)
])
optimizer=tf.keras.optimizers.legacy.Adam()
model.compile(loss="mse", optimizer=optimizer)
history = model.fit(X_train, Y_train, epochs=20,
validation_data=(X_valid, Y_valid))
Epoch 1/20
219/219 [==============================] - 0s 1ms/step - loss: 0.1342 - val_loss: 0.0637
Epoch 2/20
219/219 [==============================] - 0s 1ms/step - loss: 0.0512 - val_loss: 0.0437
Epoch 3/20
219/219 [==============================] - 0s 2ms/step - loss: 0.0390 - val_loss: 0.0360
Epoch 4/20
219/219 [==============================] - 0s 1ms/step - loss: 0.0336 - val_loss: 0.0320
Epoch 5/20
219/219 [==============================] - 0s 2ms/step - loss: 0.0305 - val_loss: 0.0294
Epoch 6/20
219/219 [==============================] - 0s 2ms/step - loss: 0.0282 - val_loss: 0.0276
Epoch 7/20
219/219 [==============================] - 0s 2ms/step - loss: 0.0265 - val_loss: 0.0260
Epoch 8/20
219/219 [==============================] - 0s 2ms/step - loss: 0.0253 - val_loss: 0.0247
Epoch 9/20
219/219 [==============================] - 0s 2ms/step - loss: 0.0242 - val_loss: 0.0238
Epoch 10/20
219/219 [==============================] - 1s 3ms/step - loss: 0.0233 - val_loss: 0.0229
Epoch 11/20
219/219 [==============================] - 0s 2ms/step - loss: 0.0225 - val_loss: 0.0222
Epoch 12/20
219/219 [==============================] - 1s 2ms/step - loss: 0.0219 - val_loss: 0.0217
Epoch 13/20
219/219 [==============================] - 1s 3ms/step - loss: 0.0214 - val_loss: 0.0212
Epoch 14/20
219/219 [==============================] - 1s 2ms/step - loss: 0.0209 - val_loss: 0.0210
Epoch 15/20
219/219 [==============================] - 1s 3ms/step - loss: 0.0205 - val_loss: 0.0205
Epoch 16/20
219/219 [==============================] - 1s 3ms/step - loss: 0.0202 - val_loss: 0.0202
Epoch 17/20
219/219 [==============================] - 1s 2ms/step - loss: 0.0198 - val_loss: 0.0197
Epoch 18/20
219/219 [==============================] - 1s 3ms/step - loss: 0.0195 - val_loss: 0.0194
Epoch 19/20
219/219 [==============================] - 1s 2ms/step - loss: 0.0192 - val_loss: 0.0191
Epoch 20/20
219/219 [==============================] - 0s 2ms/step - loss: 0.0190 - val_loss: 0.0189
Now let’s create an RNN that predicts all 10 next values at once:
np.random.seed(42)
tf.random.set_seed(42)
model = keras.models.Sequential([
keras.layers.SimpleRNN(20, return_sequences=True, input_shape=[None, 1]),
keras.layers.SimpleRNN(20),
keras.layers.Dense(10)
])
optimizer=tf.keras.optimizers.legacy.Adam()
model.compile(loss="mse", optimizer=optimizer)
history = model.fit(X_train, Y_train, epochs=20,
validation_data=(X_valid, Y_valid))
Epoch 1/20
219/219 [==============================] - 6s 24ms/step - loss: 0.0568 - val_loss: 0.0303
Epoch 2/20
219/219 [==============================] - 5s 25ms/step - loss: 0.0276 - val_loss: 0.0233
Epoch 3/20
219/219 [==============================] - 5s 24ms/step - loss: 0.0191 - val_loss: 0.0157
Epoch 4/20
219/219 [==============================] - 5s 24ms/step - loss: 0.0157 - val_loss: 0.0147
Epoch 5/20
219/219 [==============================] - 5s 24ms/step - loss: 0.0133 - val_loss: 0.0114
Epoch 6/20
219/219 [==============================] - 5s 25ms/step - loss: 0.0122 - val_loss: 0.0106
Epoch 7/20
219/219 [==============================] - 5s 23ms/step - loss: 0.0113 - val_loss: 0.0102
Epoch 8/20
219/219 [==============================] - 5s 24ms/step - loss: 0.0111 - val_loss: 0.0095
Epoch 9/20
219/219 [==============================] - 5s 24ms/step - loss: 0.0108 - val_loss: 0.0114
Epoch 10/20
219/219 [==============================] - 5s 25ms/step - loss: 0.0103 - val_loss: 0.0097
Epoch 11/20
219/219 [==============================] - 6s 25ms/step - loss: 0.0104 - val_loss: 0.0091
Epoch 12/20
219/219 [==============================] - 5s 24ms/step - loss: 0.0098 - val_loss: 0.0122
Epoch 13/20
219/219 [==============================] - 5s 24ms/step - loss: 0.0095 - val_loss: 0.0081
Epoch 14/20
219/219 [==============================] - 5s 24ms/step - loss: 0.0093 - val_loss: 0.0097
Epoch 15/20
219/219 [==============================] - 5s 25ms/step - loss: 0.0090 - val_loss: 0.0083
Epoch 16/20
219/219 [==============================] - 6s 25ms/step - loss: 0.0094 - val_loss: 0.0084
Epoch 17/20
219/219 [==============================] - 5s 24ms/step - loss: 0.0091 - val_loss: 0.0087
Epoch 18/20
219/219 [==============================] - 5s 24ms/step - loss: 0.0089 - val_loss: 0.0088
Epoch 19/20
219/219 [==============================] - 5s 25ms/step - loss: 0.0089 - val_loss: 0.0082
Epoch 20/20
219/219 [==============================] - 5s 25ms/step - loss: 0.0089 - val_loss: 0.0082
np.random.seed(43)
series = generate_time_series(1, 50 + 10)
X_new, Y_new = series[:, :50, :], series[:, -10:, :]
Y_pred = model.predict(X_new)[..., np.newaxis]
1/1 [==============================] - 0s 127ms/step
plot_multiple_forecasts(X_new, Y_new, Y_pred)
plt.show()
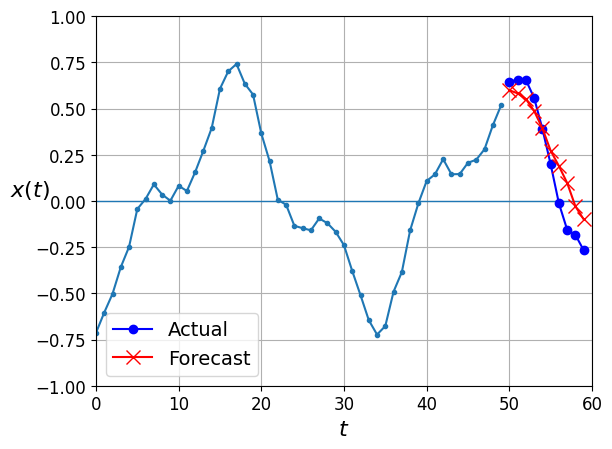
Now let’s create an RNN that predicts the next 10 steps at each time step. That is, instead of just forecasting time steps 50 to 59 based on time steps 0 to 49, it will forecast time steps 1 to 10 at time step 0, then time steps 2 to 11 at time step 1, and so on, and finally it will forecast time steps 50 to 59 at the last time step. Notice that the model is causal: when it makes predictions at any time step, it can only see past time steps.
np.random.seed(42)
n_steps = 50
series = generate_time_series(10000, n_steps + 10)
X_train = series[:7000, :n_steps]
X_valid = series[7000:9000, :n_steps]
X_test = series[9000:, :n_steps]
Y = np.empty((10000, n_steps, 10))
for step_ahead in range(1, 10 + 1):
Y[..., step_ahead - 1] = series[..., step_ahead:step_ahead + n_steps, 0]
Y_train = Y[:7000]
Y_valid = Y[7000:9000]
Y_test = Y[9000:]
X_train.shape, Y_train.shape
((7000, 50, 1), (7000, 50, 10))
np.random.seed(42)
tf.random.set_seed(42)
model = keras.models.Sequential([
keras.layers.SimpleRNN(20, return_sequences=True, input_shape=[None, 1]),
keras.layers.SimpleRNN(20, return_sequences=True),
keras.layers.TimeDistributed(keras.layers.Dense(10))
])
def last_time_step_mse(Y_true, Y_pred):
return keras.metrics.mean_squared_error(Y_true[:, -1], Y_pred[:, -1])
model.compile(loss="mse", optimizer=keras.optimizers.legacy.Adam(learning_rate=0.01), metrics=[last_time_step_mse])
history = model.fit(X_train, Y_train, epochs=20,
validation_data=(X_valid, Y_valid))
Epoch 1/20
219/219 [==============================] - 6s 25ms/step - loss: 0.0523 - last_time_step_mse: 0.0421 - val_loss: 0.0425 - val_last_time_step_mse: 0.0308
Epoch 2/20
219/219 [==============================] - 5s 24ms/step - loss: 0.0407 - last_time_step_mse: 0.0288 - val_loss: 0.0333 - val_last_time_step_mse: 0.0194
Epoch 3/20
219/219 [==============================] - 5s 24ms/step - loss: 0.0335 - last_time_step_mse: 0.0214 - val_loss: 0.0312 - val_last_time_step_mse: 0.0203
Epoch 4/20
219/219 [==============================] - 5s 25ms/step - loss: 0.0300 - last_time_step_mse: 0.0179 - val_loss: 0.0262 - val_last_time_step_mse: 0.0130
Epoch 5/20
219/219 [==============================] - 5s 24ms/step - loss: 0.0262 - last_time_step_mse: 0.0135 - val_loss: 0.0235 - val_last_time_step_mse: 0.0105
Epoch 6/20
219/219 [==============================] - 5s 25ms/step - loss: 0.0234 - last_time_step_mse: 0.0109 - val_loss: 0.0234 - val_last_time_step_mse: 0.0111
Epoch 7/20
219/219 [==============================] - 5s 24ms/step - loss: 0.0220 - last_time_step_mse: 0.0101 - val_loss: 0.0202 - val_last_time_step_mse: 0.0082
Epoch 8/20
219/219 [==============================] - 5s 25ms/step - loss: 0.0208 - last_time_step_mse: 0.0088 - val_loss: 0.0198 - val_last_time_step_mse: 0.0081
Epoch 9/20
219/219 [==============================] - 5s 23ms/step - loss: 0.0203 - last_time_step_mse: 0.0085 - val_loss: 0.0197 - val_last_time_step_mse: 0.0081
Epoch 10/20
219/219 [==============================] - 5s 23ms/step - loss: 0.0197 - last_time_step_mse: 0.0078 - val_loss: 0.0208 - val_last_time_step_mse: 0.0111
Epoch 11/20
219/219 [==============================] - 5s 23ms/step - loss: 0.0197 - last_time_step_mse: 0.0080 - val_loss: 0.0194 - val_last_time_step_mse: 0.0075
Epoch 12/20
219/219 [==============================] - 5s 24ms/step - loss: 0.0193 - last_time_step_mse: 0.0076 - val_loss: 0.0178 - val_last_time_step_mse: 0.0060
Epoch 13/20
219/219 [==============================] - 6s 25ms/step - loss: 0.0187 - last_time_step_mse: 0.0069 - val_loss: 0.0194 - val_last_time_step_mse: 0.0078
Epoch 14/20
219/219 [==============================] - 5s 25ms/step - loss: 0.0185 - last_time_step_mse: 0.0068 - val_loss: 0.0183 - val_last_time_step_mse: 0.0069
Epoch 15/20
219/219 [==============================] - 5s 25ms/step - loss: 0.0187 - last_time_step_mse: 0.0071 - val_loss: 0.0177 - val_last_time_step_mse: 0.0064
Epoch 16/20
219/219 [==============================] - 5s 24ms/step - loss: 0.0187 - last_time_step_mse: 0.0071 - val_loss: 0.0191 - val_last_time_step_mse: 0.0083
Epoch 17/20
219/219 [==============================] - 5s 25ms/step - loss: 0.0183 - last_time_step_mse: 0.0066 - val_loss: 0.0173 - val_last_time_step_mse: 0.0059
Epoch 18/20
219/219 [==============================] - 5s 24ms/step - loss: 0.0183 - last_time_step_mse: 0.0069 - val_loss: 0.0178 - val_last_time_step_mse: 0.0067
Epoch 19/20
219/219 [==============================] - 6s 25ms/step - loss: 0.0178 - last_time_step_mse: 0.0063 - val_loss: 0.0188 - val_last_time_step_mse: 0.0072
Epoch 20/20
219/219 [==============================] - 5s 25ms/step - loss: 0.0181 - last_time_step_mse: 0.0068 - val_loss: 0.0199 - val_last_time_step_mse: 0.0083
np.random.seed(43)
series = generate_time_series(1, 50 + 10)
X_new, Y_new = series[:, :50, :], series[:, 50:, :]
Y_pred = model.predict(X_new)[:, -1][..., np.newaxis]
1/1 [==============================] - 0s 127ms/step
plot_multiple_forecasts(X_new, Y_new, Y_pred)
plt.show()
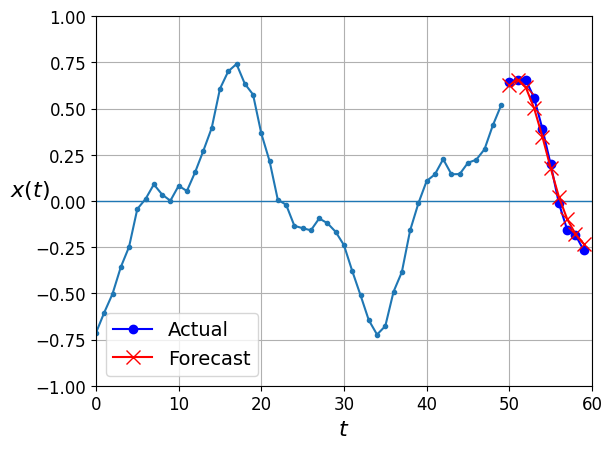
Deep RNN with Batch Norm#
np.random.seed(42)
tf.random.set_seed(42)
model = keras.models.Sequential([
keras.layers.SimpleRNN(20, return_sequences=True, input_shape=[None, 1]),
keras.layers.BatchNormalization(),
keras.layers.SimpleRNN(20, return_sequences=True),
keras.layers.BatchNormalization(),
keras.layers.TimeDistributed(keras.layers.Dense(10))
])
optimizer=tf.keras.optimizers.legacy.Adam()
model.compile(loss="mse", optimizer=optimizer, metrics=[last_time_step_mse])
history = model.fit(X_train, Y_train, epochs=20,
validation_data=(X_valid, Y_valid))
Epoch 1/20
219/219 [==============================] - 7s 26ms/step - loss: 0.1921 - last_time_step_mse: 0.1716 - val_loss: 0.0865 - val_last_time_step_mse: 0.0776
Epoch 2/20
219/219 [==============================] - 6s 25ms/step - loss: 0.0540 - last_time_step_mse: 0.0439 - val_loss: 0.0519 - val_last_time_step_mse: 0.0421
Epoch 3/20
219/219 [==============================] - 6s 26ms/step - loss: 0.0466 - last_time_step_mse: 0.0365 - val_loss: 0.0451 - val_last_time_step_mse: 0.0345
Epoch 4/20
219/219 [==============================] - 5s 25ms/step - loss: 0.0420 - last_time_step_mse: 0.0312 - val_loss: 0.0404 - val_last_time_step_mse: 0.0295
Epoch 5/20
219/219 [==============================] - 6s 26ms/step - loss: 0.0383 - last_time_step_mse: 0.0267 - val_loss: 0.0373 - val_last_time_step_mse: 0.0256
Epoch 6/20
219/219 [==============================] - 6s 25ms/step - loss: 0.0357 - last_time_step_mse: 0.0235 - val_loss: 0.0356 - val_last_time_step_mse: 0.0232
Epoch 7/20
219/219 [==============================] - 6s 25ms/step - loss: 0.0342 - last_time_step_mse: 0.0222 - val_loss: 0.0338 - val_last_time_step_mse: 0.0218
Epoch 8/20
219/219 [==============================] - 6s 25ms/step - loss: 0.0326 - last_time_step_mse: 0.0206 - val_loss: 0.0318 - val_last_time_step_mse: 0.0197
Epoch 9/20
219/219 [==============================] - 6s 26ms/step - loss: 0.0315 - last_time_step_mse: 0.0193 - val_loss: 0.0311 - val_last_time_step_mse: 0.0190
Epoch 10/20
219/219 [==============================] - 6s 26ms/step - loss: 0.0310 - last_time_step_mse: 0.0190 - val_loss: 0.0309 - val_last_time_step_mse: 0.0191
Epoch 11/20
219/219 [==============================] - 6s 26ms/step - loss: 0.0304 - last_time_step_mse: 0.0184 - val_loss: 0.0313 - val_last_time_step_mse: 0.0197
Epoch 12/20
219/219 [==============================] - 6s 26ms/step - loss: 0.0298 - last_time_step_mse: 0.0178 - val_loss: 0.0292 - val_last_time_step_mse: 0.0169
Epoch 13/20
219/219 [==============================] - 6s 26ms/step - loss: 0.0293 - last_time_step_mse: 0.0173 - val_loss: 0.0289 - val_last_time_step_mse: 0.0169
Epoch 14/20
219/219 [==============================] - 6s 25ms/step - loss: 0.0290 - last_time_step_mse: 0.0169 - val_loss: 0.0282 - val_last_time_step_mse: 0.0163
Epoch 15/20
219/219 [==============================] - 6s 25ms/step - loss: 0.0286 - last_time_step_mse: 0.0166 - val_loss: 0.0280 - val_last_time_step_mse: 0.0159
Epoch 16/20
219/219 [==============================] - 6s 26ms/step - loss: 0.0284 - last_time_step_mse: 0.0164 - val_loss: 0.0293 - val_last_time_step_mse: 0.0176
Epoch 17/20
219/219 [==============================] - 6s 25ms/step - loss: 0.0281 - last_time_step_mse: 0.0161 - val_loss: 0.0281 - val_last_time_step_mse: 0.0155
Epoch 18/20
219/219 [==============================] - 6s 26ms/step - loss: 0.0277 - last_time_step_mse: 0.0157 - val_loss: 0.0273 - val_last_time_step_mse: 0.0153
Epoch 19/20
219/219 [==============================] - 6s 26ms/step - loss: 0.0275 - last_time_step_mse: 0.0155 - val_loss: 0.0272 - val_last_time_step_mse: 0.0153
Epoch 20/20
219/219 [==============================] - 5s 25ms/step - loss: 0.0273 - last_time_step_mse: 0.0153 - val_loss: 0.0274 - val_last_time_step_mse: 0.0152
Deep RNNs with Layer Norm#
#from tensorflow.keras.layers import LayerNormalization
from keras.layers import LayerNormalization
class LNSimpleRNNCell(keras.layers.Layer):
def __init__(self, units, activation="tanh", **kwargs):
super().__init__(**kwargs)
self.state_size = units
self.output_size = units
self.simple_rnn_cell = keras.layers.SimpleRNNCell(units,
activation=None)
self.layer_norm = LayerNormalization()
self.activation = keras.activations.get(activation)
def get_initial_state(self, inputs=None, batch_size=None, dtype=None):
if inputs is not None:
batch_size = tf.shape(inputs)[0]
dtype = inputs.dtype
return [tf.zeros([batch_size, self.state_size], dtype=dtype)]
def call(self, inputs, states):
outputs, new_states = self.simple_rnn_cell(inputs, states)
norm_outputs = self.activation(self.layer_norm(outputs))
return norm_outputs, [norm_outputs]
np.random.seed(42)
tf.random.set_seed(42)
model = keras.models.Sequential([
keras.layers.RNN(LNSimpleRNNCell(20), return_sequences=True,
input_shape=[None, 1]),
keras.layers.RNN(LNSimpleRNNCell(20), return_sequences=True),
keras.layers.TimeDistributed(keras.layers.Dense(10))
])
optimizer=tf.keras.optimizers.legacy.Adam()
model.compile(loss="mse", optimizer=optimizer, metrics=[last_time_step_mse])
history = model.fit(X_train, Y_train, epochs=20,
validation_data=(X_valid, Y_valid))
Epoch 1/20
3/219 [..............................] - ETA: 9s - loss: 0.6127 - last_time_step_mse: 0.5811 219/219 [==============================] - 13s 54ms/step - loss: 0.1121 - last_time_step_mse: 0.0978 - val_loss: 0.0626 - val_last_time_step_mse: 0.0526
Epoch 2/20
219/219 [==============================] - 16s 71ms/step - loss: 0.0583 - last_time_step_mse: 0.0486 - val_loss: 0.0546 - val_last_time_step_mse: 0.0455
Epoch 3/20
219/219 [==============================] - 14s 66ms/step - loss: 0.0517 - last_time_step_mse: 0.0415 - val_loss: 0.0484 - val_last_time_step_mse: 0.0380
Epoch 4/20
219/219 [==============================] - 14s 65ms/step - loss: 0.0461 - last_time_step_mse: 0.0355 - val_loss: 0.0437 - val_last_time_step_mse: 0.0340
Epoch 5/20
219/219 [==============================] - 15s 67ms/step - loss: 0.0416 - last_time_step_mse: 0.0309 - val_loss: 0.0401 - val_last_time_step_mse: 0.0301
Epoch 6/20
219/219 [==============================] - 14s 66ms/step - loss: 0.0390 - last_time_step_mse: 0.0280 - val_loss: 0.0375 - val_last_time_step_mse: 0.0262
Epoch 7/20
219/219 [==============================] - 13s 61ms/step - loss: 0.0369 - last_time_step_mse: 0.0258 - val_loss: 0.0357 - val_last_time_step_mse: 0.0239
Epoch 8/20
219/219 [==============================] - 14s 62ms/step - loss: 0.0354 - last_time_step_mse: 0.0239 - val_loss: 0.0349 - val_last_time_step_mse: 0.0229
Epoch 9/20
219/219 [==============================] - 13s 61ms/step - loss: 0.0340 - last_time_step_mse: 0.0221 - val_loss: 0.0336 - val_last_time_step_mse: 0.0218
Epoch 10/20
219/219 [==============================] - 14s 62ms/step - loss: 0.0336 - last_time_step_mse: 0.0216 - val_loss: 0.0333 - val_last_time_step_mse: 0.0211
Epoch 11/20
219/219 [==============================] - 16s 72ms/step - loss: 0.0327 - last_time_step_mse: 0.0204 - val_loss: 0.0318 - val_last_time_step_mse: 0.0194
Epoch 12/20
219/219 [==============================] - 14s 63ms/step - loss: 0.0320 - last_time_step_mse: 0.0195 - val_loss: 0.0315 - val_last_time_step_mse: 0.0193
Epoch 13/20
219/219 [==============================] - 15s 70ms/step - loss: 0.0315 - last_time_step_mse: 0.0190 - val_loss: 0.0307 - val_last_time_step_mse: 0.0182
Epoch 14/20
219/219 [==============================] - 13s 61ms/step - loss: 0.0309 - last_time_step_mse: 0.0184 - val_loss: 0.0308 - val_last_time_step_mse: 0.0187
Epoch 15/20
219/219 [==============================] - 15s 66ms/step - loss: 0.0305 - last_time_step_mse: 0.0179 - val_loss: 0.0299 - val_last_time_step_mse: 0.0173
Epoch 16/20
219/219 [==============================] - 13s 62ms/step - loss: 0.0300 - last_time_step_mse: 0.0174 - val_loss: 0.0296 - val_last_time_step_mse: 0.0173
Epoch 17/20
219/219 [==============================] - 15s 68ms/step - loss: 0.0295 - last_time_step_mse: 0.0170 - val_loss: 0.0289 - val_last_time_step_mse: 0.0160
Epoch 18/20
219/219 [==============================] - 14s 65ms/step - loss: 0.0290 - last_time_step_mse: 0.0163 - val_loss: 0.0281 - val_last_time_step_mse: 0.0153
Epoch 19/20
219/219 [==============================] - 14s 64ms/step - loss: 0.0286 - last_time_step_mse: 0.0158 - val_loss: 0.0279 - val_last_time_step_mse: 0.0149
Epoch 20/20
219/219 [==============================] - 14s 63ms/step - loss: 0.0282 - last_time_step_mse: 0.0154 - val_loss: 0.0274 - val_last_time_step_mse: 0.0144
2023-04-04 13:15:05.308735: I tensorflow/compiler/xla/stream_executor/cuda/cuda_dnn.cc:424] Loaded cuDNN version 8600
Creating a Custom RNN Class#
class MyRNN(keras.layers.Layer):
def __init__(self, cell, return_sequences=False, **kwargs):
super().__init__(**kwargs)
self.cell = cell
self.return_sequences = return_sequences
self.get_initial_state = getattr(
self.cell, "get_initial_state", self.fallback_initial_state)
def fallback_initial_state(self, inputs):
batch_size = tf.shape(inputs)[0]
return [tf.zeros([batch_size, self.cell.state_size], dtype=inputs.dtype)]
@tf.function
def call(self, inputs):
states = self.get_initial_state(inputs)
shape = tf.shape(inputs)
batch_size = shape[0]
n_steps = shape[1]
sequences = tf.TensorArray(
inputs.dtype, size=(n_steps if self.return_sequences else 0))
outputs = tf.zeros(shape=[batch_size, self.cell.output_size], dtype=inputs.dtype)
for step in tf.range(n_steps):
outputs, states = self.cell(inputs[:, step], states)
if self.return_sequences:
sequences = sequences.write(step, outputs)
if self.return_sequences:
return tf.transpose(sequences.stack(), [1, 0, 2])
else:
return outputs
np.random.seed(42)
tf.random.set_seed(42)
model = keras.models.Sequential([
MyRNN(LNSimpleRNNCell(20), return_sequences=True,
input_shape=[None, 1]),
MyRNN(LNSimpleRNNCell(20), return_sequences=True),
keras.layers.TimeDistributed(keras.layers.Dense(10))
])
optimizer=tf.keras.optimizers.legacy.Adam()
model.compile(loss="mse", optimizer=optimizer, metrics=[last_time_step_mse])
history = model.fit(X_train, Y_train, epochs=20,
validation_data=(X_valid, Y_valid))
Epoch 1/20
219/219 [==============================] - 16s 67ms/step - loss: 0.1450 - last_time_step_mse: 0.1322 - val_loss: 0.0623 - val_last_time_step_mse: 0.0509
Epoch 2/20
219/219 [==============================] - 15s 69ms/step - loss: 0.0557 - last_time_step_mse: 0.0451 - val_loss: 0.0507 - val_last_time_step_mse: 0.0401
Epoch 3/20
219/219 [==============================] - 15s 67ms/step - loss: 0.0474 - last_time_step_mse: 0.0367 - val_loss: 0.0446 - val_last_time_step_mse: 0.0334
Epoch 4/20
219/219 [==============================] - 14s 66ms/step - loss: 0.0429 - last_time_step_mse: 0.0320 - val_loss: 0.0405 - val_last_time_step_mse: 0.0285
Epoch 5/20
219/219 [==============================] - 14s 66ms/step - loss: 0.0406 - last_time_step_mse: 0.0286 - val_loss: 0.0383 - val_last_time_step_mse: 0.0248
Epoch 6/20
219/219 [==============================] - 14s 65ms/step - loss: 0.0360 - last_time_step_mse: 0.0216 - val_loss: 0.0343 - val_last_time_step_mse: 0.0197
Epoch 7/20
219/219 [==============================] - 14s 65ms/step - loss: 0.0338 - last_time_step_mse: 0.0191 - val_loss: 0.0321 - val_last_time_step_mse: 0.0170
Epoch 8/20
219/219 [==============================] - 16s 71ms/step - loss: 0.0319 - last_time_step_mse: 0.0171 - val_loss: 0.0309 - val_last_time_step_mse: 0.0158
Epoch 9/20
219/219 [==============================] - 14s 65ms/step - loss: 0.0308 - last_time_step_mse: 0.0159 - val_loss: 0.0302 - val_last_time_step_mse: 0.0158
Epoch 10/20
219/219 [==============================] - 16s 72ms/step - loss: 0.0302 - last_time_step_mse: 0.0154 - val_loss: 0.0296 - val_last_time_step_mse: 0.0147
Epoch 11/20
219/219 [==============================] - 14s 65ms/step - loss: 0.0296 - last_time_step_mse: 0.0149 - val_loss: 0.0295 - val_last_time_step_mse: 0.0144
Epoch 12/20
219/219 [==============================] - 16s 75ms/step - loss: 0.0295 - last_time_step_mse: 0.0143 - val_loss: 0.0282 - val_last_time_step_mse: 0.0139
Epoch 13/20
219/219 [==============================] - 16s 74ms/step - loss: 0.0281 - last_time_step_mse: 0.0131 - val_loss: 0.0280 - val_last_time_step_mse: 0.0131
Epoch 14/20
219/219 [==============================] - 16s 71ms/step - loss: 0.0276 - last_time_step_mse: 0.0126 - val_loss: 0.0269 - val_last_time_step_mse: 0.0125
Epoch 15/20
219/219 [==============================] - 12s 55ms/step - loss: 0.0269 - last_time_step_mse: 0.0117 - val_loss: 0.0255 - val_last_time_step_mse: 0.0103
Epoch 16/20
219/219 [==============================] - 12s 53ms/step - loss: 0.0260 - last_time_step_mse: 0.0110 - val_loss: 0.0251 - val_last_time_step_mse: 0.0104
Epoch 17/20
219/219 [==============================] - 12s 53ms/step - loss: 0.0253 - last_time_step_mse: 0.0103 - val_loss: 0.0250 - val_last_time_step_mse: 0.0104
Epoch 18/20
219/219 [==============================] - 12s 53ms/step - loss: 0.0245 - last_time_step_mse: 0.0096 - val_loss: 0.0237 - val_last_time_step_mse: 0.0091
Epoch 19/20
219/219 [==============================] - 12s 55ms/step - loss: 0.0241 - last_time_step_mse: 0.0091 - val_loss: 0.0234 - val_last_time_step_mse: 0.0086
Epoch 20/20
219/219 [==============================] - 12s 53ms/step - loss: 0.0236 - last_time_step_mse: 0.0090 - val_loss: 0.0230 - val_last_time_step_mse: 0.0081
2023-04-04 13:19:47.603857: I tensorflow/core/common_runtime/executor.cc:1197] [/device:CPU:0] (DEBUG INFO) Executor start aborting (this does not indicate an error and you can ignore this message): INVALID_ARGUMENT: You must feed a value for placeholder tensor 'Placeholder' with dtype float and shape [?,?,1]
[[{{node Placeholder}}]]
2023-04-04 13:19:47.691018: I tensorflow/core/common_runtime/executor.cc:1197] [/device:CPU:0] (DEBUG INFO) Executor start aborting (this does not indicate an error and you can ignore this message): INVALID_ARGUMENT: You must feed a value for placeholder tensor 'Placeholder' with dtype float and shape [?,?,20]
[[{{node Placeholder}}]]
LSTMs#
np.random.seed(42)
tf.random.set_seed(42)
model = keras.models.Sequential([
keras.layers.LSTM(20, return_sequences=True, input_shape=[None, 1]),
keras.layers.LSTM(20, return_sequences=True),
keras.layers.TimeDistributed(keras.layers.Dense(10))
])
optimizer=tf.keras.optimizers.legacy.Adam()
model.compile(loss="mse", optimizer=optimizer, metrics=[last_time_step_mse])
history = model.fit(X_train, Y_train, epochs=20,
validation_data=(X_valid, Y_valid))
Epoch 1/20
215/219 [============================>.] - ETA: 0s - loss: 0.0803 - last_time_step_mse: 0.0660219/219 [==============================] - 4s 12ms/step - loss: 0.0798 - last_time_step_mse: 0.0655 - val_loss: 0.0553 - val_last_time_step_mse: 0.0368
Epoch 2/20
219/219 [==============================] - 2s 9ms/step - loss: 0.0467 - last_time_step_mse: 0.0266 - val_loss: 0.0400 - val_last_time_step_mse: 0.0198
Epoch 3/20
219/219 [==============================] - 2s 9ms/step - loss: 0.0373 - last_time_step_mse: 0.0171 - val_loss: 0.0357 - val_last_time_step_mse: 0.0161
Epoch 4/20
219/219 [==============================] - 2s 10ms/step - loss: 0.0342 - last_time_step_mse: 0.0147 - val_loss: 0.0330 - val_last_time_step_mse: 0.0133
Epoch 5/20
219/219 [==============================] - 2s 10ms/step - loss: 0.0321 - last_time_step_mse: 0.0135 - val_loss: 0.0313 - val_last_time_step_mse: 0.0132
Epoch 6/20
219/219 [==============================] - 2s 10ms/step - loss: 0.0304 - last_time_step_mse: 0.0120 - val_loss: 0.0294 - val_last_time_step_mse: 0.0110
Epoch 7/20
219/219 [==============================] - 2s 9ms/step - loss: 0.0291 - last_time_step_mse: 0.0112 - val_loss: 0.0284 - val_last_time_step_mse: 0.0111
Epoch 8/20
219/219 [==============================] - 2s 10ms/step - loss: 0.0281 - last_time_step_mse: 0.0104 - val_loss: 0.0274 - val_last_time_step_mse: 0.0101
Epoch 9/20
219/219 [==============================] - 2s 10ms/step - loss: 0.0273 - last_time_step_mse: 0.0102 - val_loss: 0.0269 - val_last_time_step_mse: 0.0099
Epoch 10/20
219/219 [==============================] - 2s 9ms/step - loss: 0.0268 - last_time_step_mse: 0.0101 - val_loss: 0.0264 - val_last_time_step_mse: 0.0098
Epoch 11/20
219/219 [==============================] - 2s 8ms/step - loss: 0.0263 - last_time_step_mse: 0.0097 - val_loss: 0.0259 - val_last_time_step_mse: 0.0093
Epoch 12/20
219/219 [==============================] - 2s 10ms/step - loss: 0.0258 - last_time_step_mse: 0.0095 - val_loss: 0.0259 - val_last_time_step_mse: 0.0100
Epoch 13/20
219/219 [==============================] - 2s 9ms/step - loss: 0.0254 - last_time_step_mse: 0.0093 - val_loss: 0.0251 - val_last_time_step_mse: 0.0093
Epoch 14/20
219/219 [==============================] - 2s 9ms/step - loss: 0.0251 - last_time_step_mse: 0.0092 - val_loss: 0.0249 - val_last_time_step_mse: 0.0090
Epoch 15/20
219/219 [==============================] - 2s 9ms/step - loss: 0.0247 - last_time_step_mse: 0.0090 - val_loss: 0.0247 - val_last_time_step_mse: 0.0086
Epoch 16/20
219/219 [==============================] - 2s 9ms/step - loss: 0.0245 - last_time_step_mse: 0.0091 - val_loss: 0.0244 - val_last_time_step_mse: 0.0090
Epoch 17/20
219/219 [==============================] - 2s 10ms/step - loss: 0.0241 - last_time_step_mse: 0.0088 - val_loss: 0.0242 - val_last_time_step_mse: 0.0091
Epoch 18/20
219/219 [==============================] - 2s 9ms/step - loss: 0.0238 - last_time_step_mse: 0.0087 - val_loss: 0.0235 - val_last_time_step_mse: 0.0081
Epoch 19/20
219/219 [==============================] - 2s 9ms/step - loss: 0.0235 - last_time_step_mse: 0.0086 - val_loss: 0.0236 - val_last_time_step_mse: 0.0092
Epoch 20/20
219/219 [==============================] - 2s 9ms/step - loss: 0.0232 - last_time_step_mse: 0.0084 - val_loss: 0.0233 - val_last_time_step_mse: 0.0080
2023-04-04 13:24:29.090311: I tensorflow/core/common_runtime/executor.cc:1197] [/device:CPU:0] (DEBUG INFO) Executor start aborting (this does not indicate an error and you can ignore this message): INVALID_ARGUMENT: You must feed a value for placeholder tensor 'gradients/split_2_grad/concat/split_2/split_dim' with dtype int32
[[{{node gradients/split_2_grad/concat/split_2/split_dim}}]]
2023-04-04 13:24:29.091362: I tensorflow/core/common_runtime/executor.cc:1197] [/device:CPU:0] (DEBUG INFO) Executor start aborting (this does not indicate an error and you can ignore this message): INVALID_ARGUMENT: You must feed a value for placeholder tensor 'gradients/split_grad/concat/split/split_dim' with dtype int32
[[{{node gradients/split_grad/concat/split/split_dim}}]]
2023-04-04 13:24:29.092012: I tensorflow/core/common_runtime/executor.cc:1197] [/device:CPU:0] (DEBUG INFO) Executor start aborting (this does not indicate an error and you can ignore this message): INVALID_ARGUMENT: You must feed a value for placeholder tensor 'gradients/split_1_grad/concat/split_1/split_dim' with dtype int32
[[{{node gradients/split_1_grad/concat/split_1/split_dim}}]]
2023-04-04 13:24:29.192498: I tensorflow/core/common_runtime/executor.cc:1197] [/device:CPU:0] (DEBUG INFO) Executor start aborting (this does not indicate an error and you can ignore this message): INVALID_ARGUMENT: You must feed a value for placeholder tensor 'gradients/split_2_grad/concat/split_2/split_dim' with dtype int32
[[{{node gradients/split_2_grad/concat/split_2/split_dim}}]]
2023-04-04 13:24:29.193364: I tensorflow/core/common_runtime/executor.cc:1197] [/device:CPU:0] (DEBUG INFO) Executor start aborting (this does not indicate an error and you can ignore this message): INVALID_ARGUMENT: You must feed a value for placeholder tensor 'gradients/split_grad/concat/split/split_dim' with dtype int32
[[{{node gradients/split_grad/concat/split/split_dim}}]]
2023-04-04 13:24:29.194040: I tensorflow/core/common_runtime/executor.cc:1197] [/device:CPU:0] (DEBUG INFO) Executor start aborting (this does not indicate an error and you can ignore this message): INVALID_ARGUMENT: You must feed a value for placeholder tensor 'gradients/split_1_grad/concat/split_1/split_dim' with dtype int32
[[{{node gradients/split_1_grad/concat/split_1/split_dim}}]]
2023-04-04 13:24:29.389301: I tensorflow/core/common_runtime/executor.cc:1197] [/device:CPU:0] (DEBUG INFO) Executor start aborting (this does not indicate an error and you can ignore this message): INVALID_ARGUMENT: You must feed a value for placeholder tensor 'gradients/split_2_grad/concat/split_2/split_dim' with dtype int32
[[{{node gradients/split_2_grad/concat/split_2/split_dim}}]]
2023-04-04 13:24:29.390136: I tensorflow/core/common_runtime/executor.cc:1197] [/device:CPU:0] (DEBUG INFO) Executor start aborting (this does not indicate an error and you can ignore this message): INVALID_ARGUMENT: You must feed a value for placeholder tensor 'gradients/split_grad/concat/split/split_dim' with dtype int32
[[{{node gradients/split_grad/concat/split/split_dim}}]]
2023-04-04 13:24:29.391227: I tensorflow/core/common_runtime/executor.cc:1197] [/device:CPU:0] (DEBUG INFO) Executor start aborting (this does not indicate an error and you can ignore this message): INVALID_ARGUMENT: You must feed a value for placeholder tensor 'gradients/split_1_grad/concat/split_1/split_dim' with dtype int32
[[{{node gradients/split_1_grad/concat/split_1/split_dim}}]]
2023-04-04 13:24:29.502526: I tensorflow/core/common_runtime/executor.cc:1197] [/device:CPU:0] (DEBUG INFO) Executor start aborting (this does not indicate an error and you can ignore this message): INVALID_ARGUMENT: You must feed a value for placeholder tensor 'gradients/split_2_grad/concat/split_2/split_dim' with dtype int32
[[{{node gradients/split_2_grad/concat/split_2/split_dim}}]]
2023-04-04 13:24:29.503596: I tensorflow/core/common_runtime/executor.cc:1197] [/device:CPU:0] (DEBUG INFO) Executor start aborting (this does not indicate an error and you can ignore this message): INVALID_ARGUMENT: You must feed a value for placeholder tensor 'gradients/split_grad/concat/split/split_dim' with dtype int32
[[{{node gradients/split_grad/concat/split/split_dim}}]]
2023-04-04 13:24:29.504377: I tensorflow/core/common_runtime/executor.cc:1197] [/device:CPU:0] (DEBUG INFO) Executor start aborting (this does not indicate an error and you can ignore this message): INVALID_ARGUMENT: You must feed a value for placeholder tensor 'gradients/split_1_grad/concat/split_1/split_dim' with dtype int32
[[{{node gradients/split_1_grad/concat/split_1/split_dim}}]]
2023-04-04 13:24:29.936053: I tensorflow/core/common_runtime/executor.cc:1197] [/device:CPU:0] (DEBUG INFO) Executor start aborting (this does not indicate an error and you can ignore this message): INVALID_ARGUMENT: You must feed a value for placeholder tensor 'gradients/split_2_grad/concat/split_2/split_dim' with dtype int32
[[{{node gradients/split_2_grad/concat/split_2/split_dim}}]]
2023-04-04 13:24:29.937077: I tensorflow/core/common_runtime/executor.cc:1197] [/device:CPU:0] (DEBUG INFO) Executor start aborting (this does not indicate an error and you can ignore this message): INVALID_ARGUMENT: You must feed a value for placeholder tensor 'gradients/split_grad/concat/split/split_dim' with dtype int32
[[{{node gradients/split_grad/concat/split/split_dim}}]]
2023-04-04 13:24:29.937905: I tensorflow/core/common_runtime/executor.cc:1197] [/device:CPU:0] (DEBUG INFO) Executor start aborting (this does not indicate an error and you can ignore this message): INVALID_ARGUMENT: You must feed a value for placeholder tensor 'gradients/split_1_grad/concat/split_1/split_dim' with dtype int32
[[{{node gradients/split_1_grad/concat/split_1/split_dim}}]]
2023-04-04 13:24:30.048650: I tensorflow/core/common_runtime/executor.cc:1197] [/device:CPU:0] (DEBUG INFO) Executor start aborting (this does not indicate an error and you can ignore this message): INVALID_ARGUMENT: You must feed a value for placeholder tensor 'gradients/split_2_grad/concat/split_2/split_dim' with dtype int32
[[{{node gradients/split_2_grad/concat/split_2/split_dim}}]]
2023-04-04 13:24:30.049493: I tensorflow/core/common_runtime/executor.cc:1197] [/device:CPU:0] (DEBUG INFO) Executor start aborting (this does not indicate an error and you can ignore this message): INVALID_ARGUMENT: You must feed a value for placeholder tensor 'gradients/split_grad/concat/split/split_dim' with dtype int32
[[{{node gradients/split_grad/concat/split/split_dim}}]]
2023-04-04 13:24:30.050288: I tensorflow/core/common_runtime/executor.cc:1197] [/device:CPU:0] (DEBUG INFO) Executor start aborting (this does not indicate an error and you can ignore this message): INVALID_ARGUMENT: You must feed a value for placeholder tensor 'gradients/split_1_grad/concat/split_1/split_dim' with dtype int32
[[{{node gradients/split_1_grad/concat/split_1/split_dim}}]]
2023-04-04 13:24:32.964493: I tensorflow/core/common_runtime/executor.cc:1197] [/device:CPU:0] (DEBUG INFO) Executor start aborting (this does not indicate an error and you can ignore this message): INVALID_ARGUMENT: You must feed a value for placeholder tensor 'gradients/split_2_grad/concat/split_2/split_dim' with dtype int32
[[{{node gradients/split_2_grad/concat/split_2/split_dim}}]]
2023-04-04 13:24:32.965565: I tensorflow/core/common_runtime/executor.cc:1197] [/device:CPU:0] (DEBUG INFO) Executor start aborting (this does not indicate an error and you can ignore this message): INVALID_ARGUMENT: You must feed a value for placeholder tensor 'gradients/split_grad/concat/split/split_dim' with dtype int32
[[{{node gradients/split_grad/concat/split/split_dim}}]]
2023-04-04 13:24:32.966267: I tensorflow/core/common_runtime/executor.cc:1197] [/device:CPU:0] (DEBUG INFO) Executor start aborting (this does not indicate an error and you can ignore this message): INVALID_ARGUMENT: You must feed a value for placeholder tensor 'gradients/split_1_grad/concat/split_1/split_dim' with dtype int32
[[{{node gradients/split_1_grad/concat/split_1/split_dim}}]]
2023-04-04 13:24:33.077266: I tensorflow/core/common_runtime/executor.cc:1197] [/device:CPU:0] (DEBUG INFO) Executor start aborting (this does not indicate an error and you can ignore this message): INVALID_ARGUMENT: You must feed a value for placeholder tensor 'gradients/split_2_grad/concat/split_2/split_dim' with dtype int32
[[{{node gradients/split_2_grad/concat/split_2/split_dim}}]]
2023-04-04 13:24:33.078008: I tensorflow/core/common_runtime/executor.cc:1197] [/device:CPU:0] (DEBUG INFO) Executor start aborting (this does not indicate an error and you can ignore this message): INVALID_ARGUMENT: You must feed a value for placeholder tensor 'gradients/split_grad/concat/split/split_dim' with dtype int32
[[{{node gradients/split_grad/concat/split/split_dim}}]]
2023-04-04 13:24:33.078737: I tensorflow/core/common_runtime/executor.cc:1197] [/device:CPU:0] (DEBUG INFO) Executor start aborting (this does not indicate an error and you can ignore this message): INVALID_ARGUMENT: You must feed a value for placeholder tensor 'gradients/split_1_grad/concat/split_1/split_dim' with dtype int32
[[{{node gradients/split_1_grad/concat/split_1/split_dim}}]]
model.evaluate(X_valid, Y_valid)
63/63 [==============================] - 0s 5ms/step - loss: 0.0233 - last_time_step_mse: 0.0080
[0.02327839285135269, 0.008030006662011147]
plot_learning_curves(history.history["loss"], history.history["val_loss"])
plt.show()
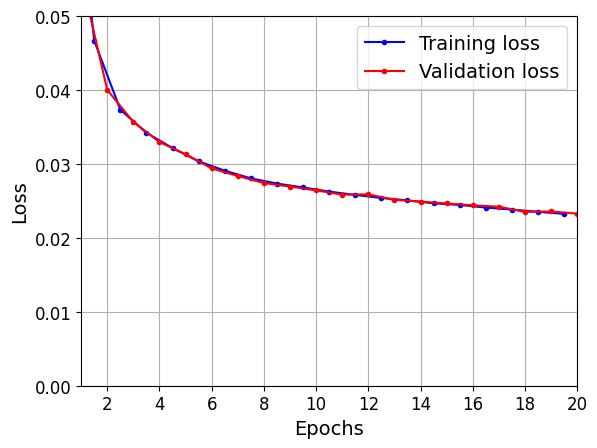
np.random.seed(43)
series = generate_time_series(1, 50 + 10)
X_new, Y_new = series[:, :50, :], series[:, 50:, :]
Y_pred = model.predict(X_new)[:, -1][..., np.newaxis]
1/1 [==============================] - 0s 335ms/step
2023-04-04 13:25:13.175645: I tensorflow/core/common_runtime/executor.cc:1197] [/device:CPU:0] (DEBUG INFO) Executor start aborting (this does not indicate an error and you can ignore this message): INVALID_ARGUMENT: You must feed a value for placeholder tensor 'gradients/split_2_grad/concat/split_2/split_dim' with dtype int32
[[{{node gradients/split_2_grad/concat/split_2/split_dim}}]]
2023-04-04 13:25:13.176571: I tensorflow/core/common_runtime/executor.cc:1197] [/device:CPU:0] (DEBUG INFO) Executor start aborting (this does not indicate an error and you can ignore this message): INVALID_ARGUMENT: You must feed a value for placeholder tensor 'gradients/split_grad/concat/split/split_dim' with dtype int32
[[{{node gradients/split_grad/concat/split/split_dim}}]]
2023-04-04 13:25:13.177468: I tensorflow/core/common_runtime/executor.cc:1197] [/device:CPU:0] (DEBUG INFO) Executor start aborting (this does not indicate an error and you can ignore this message): INVALID_ARGUMENT: You must feed a value for placeholder tensor 'gradients/split_1_grad/concat/split_1/split_dim' with dtype int32
[[{{node gradients/split_1_grad/concat/split_1/split_dim}}]]
2023-04-04 13:25:13.284336: I tensorflow/core/common_runtime/executor.cc:1197] [/device:CPU:0] (DEBUG INFO) Executor start aborting (this does not indicate an error and you can ignore this message): INVALID_ARGUMENT: You must feed a value for placeholder tensor 'gradients/split_2_grad/concat/split_2/split_dim' with dtype int32
[[{{node gradients/split_2_grad/concat/split_2/split_dim}}]]
2023-04-04 13:25:13.285894: I tensorflow/core/common_runtime/executor.cc:1197] [/device:CPU:0] (DEBUG INFO) Executor start aborting (this does not indicate an error and you can ignore this message): INVALID_ARGUMENT: You must feed a value for placeholder tensor 'gradients/split_grad/concat/split/split_dim' with dtype int32
[[{{node gradients/split_grad/concat/split/split_dim}}]]
2023-04-04 13:25:13.286792: I tensorflow/core/common_runtime/executor.cc:1197] [/device:CPU:0] (DEBUG INFO) Executor start aborting (this does not indicate an error and you can ignore this message): INVALID_ARGUMENT: You must feed a value for placeholder tensor 'gradients/split_1_grad/concat/split_1/split_dim' with dtype int32
[[{{node gradients/split_1_grad/concat/split_1/split_dim}}]]
plot_multiple_forecasts(X_new, Y_new, Y_pred)
plt.show()
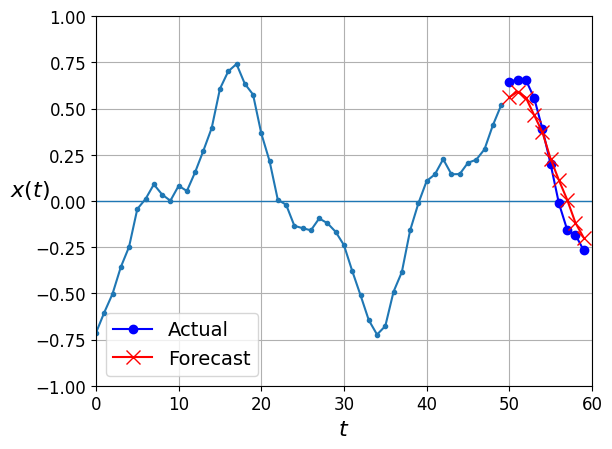
The Kernel crashed while executing code in the the current cell or a previous cell. Please review the code in the cell(s) to identify a possible cause of the failure. Click <a href='https://aka.ms/vscodeJupyterKernelCrash'>here</a> for more info. View Jupyter <a href='command:jupyter.viewOutput'>log</a> for further details.